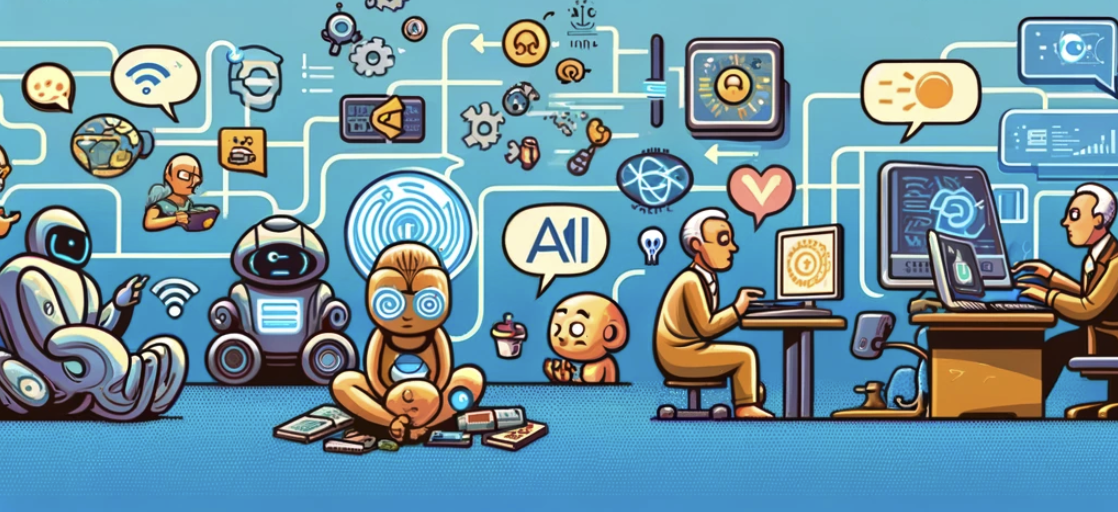
The ethics of AI and machine learning (ML) is a rapidly growing and crucial field related to the philosophy of technology.
Here’s a breakdown of key areas:
Table of Contents
1. Bias and Fairness
- Biased Data, Biased Outcomes: ML models learn from data. If training data reflects existing societal biases (racial, gender, etc.), the model will perpetuate them. This can lead to discriminatory outcomes in areas like hiring, lending, and criminal justice.
- Addressing Bias: De-biasing datasets, using diverse development teams, and rigorous fairness testing are essential. There’s a debate between focusing on technical fixes vs. acknowledging that societal bias needs broader structural solutions.
2. Transparency and Explainability (XAI)
- The Black Box Problem: Complex AI systems, like deep neural networks, can produce outputs that are difficult for humans to understand. This is a problem in high-stakes domains where decisions have significant consequences.
- The Need for Explainability: Explainable AI (XAI) aims to create models that provide insights into their decision-making processes. This is crucial for accountability, debugging, trust, and legal compliance.
3. Accountability and Responsibility
- Blurred Lines of Responsibility: Who is responsible if an AI system causes harm? The programmer, the company using it, the data provider? Legal and ethical frameworks are playing catch-up.
- Human Oversight: Especially in crucial domains, ensuring that human judgment remains central rather than blindly trusting AI outputs is a key ethical principle.
4. Privacy and Surveillance
- Data Collection and Use: AI systems are often data-hungry. Balancing the potential benefits with respect for individual privacy and preventing unauthorized data use is essential.
- AI-Powered Surveillance: Facial recognition and behavior analysis raise concerns about erosion of privacy and potential for discriminatory targeting by governments or corporations.
5. Social and Economic Impact
- Job Displacement: AI-driven automation poses risks to many jobs. Ethical considerations involve planning for just transitions, skill retraining, and potentially exploring social safety nets like universal basic income.
- Exacerbating Inequality: If the benefits of AI accrue to a small elite, it can worsen existing wealth disparities. There’s a need for policies to ensure equitable distribution of gains.
6. Weaponization of AI
- Autonomous Weapons: Lethal autonomous weapons raise profound ethical questions about machines making life-and-death decisions without human intervention. Many call for preemptive international bans.
- Manipulation and Disinformation: AI-powered deepfakes and social bots threaten to destabilize trust and erode public discourse.
7. Robot Rights and AI Personhood
- A Distant but Important Debate: As AI becomes more sophisticated, questions about potential robot sentience and whether they deserve rights surface. Most agree this is far-fetched currently, but it highlights the need for philosophical frameworks as the technology progresses.
The Importance of Interdisciplinary Approach
Addressing the ethics of AI & ML can’t be just a technical problem. It requires:
- Collaboration between computer scientists, ethicists, social scientists, and policymakers.
- Proactive ethical frameworks rather than reacting to problems after the fact.
- Public awareness and engagement to guide responsible use and regulation of AI.
Q&A – Ethics of AI & Machine Learning
What are the key ethical concerns associated with AI and machine learning?
The key ethical concerns with AI and machine learning include bias and fairness, transparency and explainability, privacy and data security, accountability, and the impact on employment. These technologies can inadvertently perpetuate existing biases, make decisions that are difficult to understand or challenge, compromise personal privacy, and disrupt job markets.
How can bias in AI and machine learning be identified and mitigated?
Bias can be identified through rigorous testing and validation against diverse data sets, including those specifically designed to uncover bias. Mitigation strategies include diversifying training data, employing algorithms that are transparent and explainable, involving multidisciplinary teams in the development process to identify potential biases, and continuously monitoring and updating AI systems to address biases as they are found.
What are the implications of AI and machine learning on privacy and data security?
AI and machine learning can pose significant risks to privacy and data security by enabling the collection, analysis, and sharing of personal data at unprecedented scales and speeds. This raises concerns about consent, data protection, and the potential for surveillance and data breaches. Ensuring robust data protection measures, transparency about data use, and adherence to privacy regulations are critical.
How do AI and machine learning impact employment and job displacement?
AI and machine learning can lead to job displacement by automating tasks previously performed by humans. While they also create new jobs and industries, the transition can be challenging for those whose skills are made redundant. Policies for retraining, education, and social support are essential to help workers adapt to the changing job landscape.
What measures can be taken to ensure transparency and accountability in AI systems?
To ensure transparency, developers can implement explainable AI techniques that make the decision-making process of AI systems understandable to humans. Accountability can be promoted through clear guidelines on the ethical use of AI, establishing oversight mechanisms, and creating legal frameworks that hold developers and users accountable for the outcomes of AI systems.
How can AI and machine learning be used ethically in decision-making processes?
Using AI and machine learning ethically in decision-making involves ensuring that the systems are fair, transparent, and accountable, do not infringe on privacy rights, and are used in ways that consider the broader societal impact. Involving stakeholders in the development process and adhering to ethical guidelines and standards can also help ensure ethical use.
What are the challenges in regulating AI and machine learning to ensure ethical use?
Regulating AI and machine learning presents challenges such as keeping pace with rapid technological advancements, addressing the global nature of technology development and deployment, ensuring regulations do not stifle innovation, and balancing technical, ethical, and legal considerations in developing regulations that are both effective and flexible.
How can the potential for AI and machine learning to perpetuate or exacerbate social inequalities be addressed?
Addressing the potential for AI to perpetuate social inequalities involves actively working to eliminate bias in AI systems, ensuring diverse representation in AI development teams, and creating AI applications that specifically aim to reduce inequalities. Policies and initiatives that promote equal access to AI technologies and their benefits are also crucial.
What role do ethics play in the development and deployment of AI and machine learning technologies?
Ethics play a critical role in guiding the responsible development and deployment of AI and machine learning technologies. Ethical considerations help ensure that these technologies are developed and used in ways that respect human rights, promote fairness, protect privacy, and contribute positively to society.
How can individuals and organizations ensure that AI and machine learning are used for societal benefit?
Individuals and organizations can ensure AI and machine learning are used for societal benefit by prioritizing ethical considerations in the development and deployment of these technologies, engaging with diverse stakeholders to understand societal needs, and focusing on applications that address societal challenges. Supporting policies and initiatives that promote the responsible use of AI for the public good is also key.